Dr Gitanjaly Chhabra, Assistant Professor at University Canada West and Prihana Vasishta, Senior Research Fellow at Punjab Engineering College, explain that biases within the banking sector can be removed with an amalgamation of humans and AI.
In the contemporary banking sector, the integration of Artificial Intelligence (AI) has revolutionised operations, providing efficiency and precision in decision-making processes. AI-driven algorithms are significantly optimising services, from customer support to risk management, offering speed and accuracy.
For instance, chatbots are being used for customer identification and authentication to provide personalised services.
Dr Hamed Taherdoost, the founder of the Hamta Business Corporation and Associate Professor at University Canada West, Vancouver, says: “AI-driven credit assessment in the banking industry has undeniably improved operational efficiency and customer experience.”
Moreover, according to the Global Payments Report 2023, “cash share of global point-of-sale (POS) is 16%,” which is estimated to be “less than 10% by 2026.” With this increase, FinTech disruptive technologies increase the responsibility to monitor AI systems.
Biases of AI in banking
As people embrace digital banking, the onus of customer satisfaction pivots on humans and machines collaboratively.
However, the growing dependence on AI in banking raises concerns about biases inherent in Machine Learning models. AI acts both like a mirror and a magnifying glass, spotlighting and amplifying the biases. Consequently, there is a distortion of judgment. Fragmented and inadequate datasets frequently result in AI ‘hallucinating’ and refraining from working efficiently.
The unfairness or potential harm caused by skewed data in AI systems is known as algorithmic bias. In the banking sector, these algorithms are often used to determine creditworthiness, assess loan applications, and detect fraudulent activities.
However, if the training data used is biased, it can lead to AI systems perpetuating existing biases. For example, a bank’s credit assessment model that primarily relies on credit score data considers two individuals, wherein X has a credit score of 720, which is considered good, and has a stable income. On the other hand, Y has a credit score of 660, which is slightly lower and has a less predictable income due to irregular freelance work. At first glance, the AI model may favour X due to a higher credit score.
However, the AI model might not take into account the context surrounding Y’s lower credit score. It could be attributed to factors such as medical bills incurred during a health crisis or student loan debt, which are not indicative of Y’s current financial stability. If the model were to consider Y’s unique circumstances, it might recognise that Y is financially responsible despite the credit score making Y eligible for a fair loan or credit assessment. Hence, if the risks of biases are not mitigated, the digital banking systems can be in jeopardy and can have adverse impacts on the banking industry.
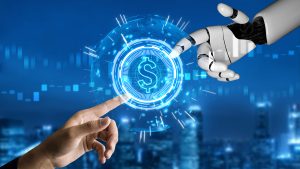
The role of human judgement in the banking sector
While AI can process vast amounts of data quickly, it lacks the ethical reasoning and contextual understanding that humans possess. To address these biases, human judgment plays a vital role in the banking sector.
Humans can recognise when a decision seems unfair, understand the broader socioeconomic context, and apply a more comprehensive set of factors in decision-making. Human intervention can help ensure that AI algorithms do not make unfair decisions or inadvertently discriminate against particular groups.
For instance, if previous mortgage lending practices were discriminatory, an AI algorithm trained on that data may continue to unfairly deny loans to specific populations. This can result in discrimination, reduced access to financial services, and, ultimately, economic inequality. In that case, through human discernment, the AI bias can be reduced.
Human and AI amalgamation is essential
Despite the essential role of human judgment in mitigating AI biases, it is vital to acknowledge the limitations. Human decisions are also prone to biases, subjective interpretations, and errors. As a result, striking a balance between AI-driven decision-making and human intervention is critical. This balance necessitates ensuring diverse datasets, continuous monitoring, adopting eXplainable AI (XAI) and including diverse teams to develop systems efficiently.
“The amalgamation of human expertise with AI’s data-driven insights present a promising approach to enhance credit evaluation, mitigating both AI hallucination risks and human biases, ultimately leading to more optimised and ethically sound decisions in the financial sector,” said Dr Taherdoost, an award-winning leader and R&D professional.
Human and AI amalgamation will augment FinTech to debias AI-models by identifying errors, ensuring well-regulated algorithmic impacts.
Further, by optimising AI-models it will lessen operational risks and enhance strategic initiatives. With expeditious digital transformation, it is crucial to continually monitor AI systems and assess their outputs. This holistic progressive transformation of the banking sector requires AI and human collaboration to remove discrepancies providing fair decision-making systems.