Vincent Huynh, Director of Data Infrastructure at QuantCube Technology, explains that data projects must be delivered using the right platforms and tools.
Businesses today want to do more with data as the benefits of data-led digital transformation become increasingly apparent across industries. Data is the lifeblood of innovation, so it’s no wonder that the interest in data tools, platforms, and advanced data management capabilities is growing—as is the employability of data science talent.
But to supercharge innovation with data, the right infrastructure and approach must be adopted to ensure success and scalability.
We all want more data, just like we want more money. But would you know what to do if $100bn was delivered to your house in change?
The challenges of managing data are numerous, from effective storage to the computing power required to process, analyse, and derive insights.
Further, it is increasingly vital to ensure all of these components are delivered within a cost-effective and sustainable model, as the overheads and energy demands associated with managing complex data infrastructure can quickly spiral, especially when there is a need to scale up.
Harnessing the power of the cloud
Running large data projects at scale requires a significant amount of computational power, so adopting the right data platforms and data engineering tools is essential for reducing costs and delivering efficiency.
When analysing terabytes of data, for example, the computational resources may require many hundreds of servers. Of course, running this many servers simultaneously over several days will quickly see costs spiral, so adopting a more sustainable approach is required.
Any organisation requiring to conduct data analysis on vast quantities of data will either require hundreds of on-premises servers, or will adopt a virtualisation model in which servers are managed as service. This cloud-based model not only delivers flexibility and scalability, but is also more cost-effective and sustainable.
By spinning up servers as they are required, for either production or development environments, for example, it’s possible to reduce the amount of administration as well as the energy used for each workflow, and enable the flexibility to allocate resources where they are needed at any time.
Big data requires a huge amount of storage. Cloud services also offer the scalability required for secure, reliable, and unlimited storage. From a business continuity and resilience perspective, cloud data centres provide confidence that projects will not grind to a halt if there is a problem at one site, as back-ups will quickly take over in the event of a failure.
With data centres significantly contributing to global carbon emissions, harnessing the power of major cloud providers that have invested heavily in technologies that reduce emissions offers a means of meeting ESG targets.
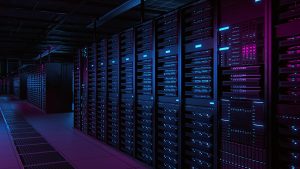
Infrastructure management
When working with big data, it’s likely that hundreds of sources of data, represented in multiple formats, will need integration so that they can be analysed effectively. By harnessing tools such as Terraform, it is possible to create script and infrastructure templates that can be reused with new data projects, vastly reducing the time needed to deliver results.
For example, a script can be developed for the purpose of analysing satellite data across a certain region to understand urban growth, which may then be easily applied to another region or geographical context. Of course, there will be some tweaking, for example, considering the unique considerations and availability of data across different geographies, but the innovation of new indicators is significantly accelerated.
The same principle works for entirely new indicators, too. If we take the above example of an indicator that analyses satellite data for urban growth across a specific region, much of the same workflow, i.e. template, can be applied to other indicators that use satellite data, such as one that focusses on deforestation.
This adds another element of automation and helps organisations analysing similar data sets across projects to establish new workflows and environments quickly and to get underway.
Expertise and data talent
Of course, an essential component in successfully delivering large data projects is talent. For each use case, subject matter expertise will be crucial to understanding the required data and validating the use case.
For example, a data project that deals with economic data should have its methodology verified and, ideally, constructed with the help of an economics expert, as the end result must be validated against a robust methodology—which also assures users, who may be paying for the insights delivered.
Big data projects today also require multidisciplined data teams to deal with both structured and unstructured data, which require a variety of expertise to manage. Having the skills to manage a data warehouse, such as SQL, alongside a data lake for object storage, such as Amazon S3, and also being able to merge large amounts of unstructured data with smaller sets of structured data is crucial for delivering an advanced data project.
These are the core fundamentals of delivering a data project at scale, while ensuring each area of development and delivery is optimised. Reducing rework and eliminating infrastructure surplus to requirements also has the added benefits of reducing operational costs and environmental impact.
For these reasons, it’s always worth looking at where optimisation of the processes and infrastructure mentioned in this article might deliver valuable results.