Richard Davies, UK Managing Director at Netcompany, discusses how businesses can leverage retrieval-augmented generation to protect their data from security risks posed by generative AI.
With deepening aspirations to embrace generative AI, controlling and managing data will be essential for businesses to create systems that deliver value without compromising safety. However, concerns about the vulnerability of data remain a fear for many businesses and their customers.
In a recent report that assessed concerns about generative AI, it found that organisations were most worried about data privacy and cyber issues (65%), employees making decisions based on inaccurate information (60%) and employee misuse and ethical risks (55%). But these fears do not have to squash AI innovation.
Retrieval-augmented generation (RAG) tools can be an excellent intermediary between business processes and AI models, with their ability to sift and fine-tune the input and output of Large Language Models (LLMs).
Think of it like a sandbox with a filter on what objects are allowed inside. RAG tools enable employees to pull from information on the inside that is catered to their organisational processes – whilst safely drawing from innovative AI models on the outside.
So, what should businesses be aware of when implementing RAG tools for generative AI, and what are its benefits for ensuring security and privacy?
Putting the workforce first
Businesses must think strategically in the early stages of AI implementation to prevent unnecessary risks. Part of that prevention is establishing early communication with employees about how and where they might find generative AI useful.
Workforces are already experimenting with AI tools, so businesses cannot afford to act too late.
If guidance is not in place, individuals will naturally put themselves – and the business – at risk without knowing it. Security should be easy and accessible, too, especially since 66% of employees say they prioritise daily tasks over cybersecurity.
RAG can be integrated within business systems to exist alongside current tools and processes. No one wants the added inconvenience of switching back and forth to get an answer. AI should enhance productivity, not hinder it.
Establishing ownership and trust
Collaboration is an essential factor for making the use of generative AI safe, whether you use RAG or not. The action of just one individual can sometimes shatter plans to maintain security – the famous case being when Samsung employees uploaded confidential code to ChatGPT, accidentally leaking trade secrets.
When adopting generative AI, complying with the General Data Protection Regulation (GDPR) should be an integral part.
Businesses should know how their data is being processed by AI, where it is being stored and who has access to it. This should also be embedded into the internal responsibilities allocated within the business.
Organisations should establish a framework that prioritises things such as privacy in data management, the active provision of employee education and training, and the minimisation of unnecessary stored data.
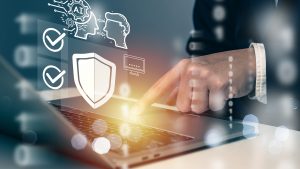
Businesses must quickly establish what kinds of data they have and who should own what. Data owners can help determine which information is and isn’t sensitive and advise which data can be declassified. That might mean redacting names, places, company names, etc.
Reinforcing LLMs with a retrieval-augmented generation tool that can identify the sensitive data unique to your business can even allow for deploying sophisticated algorithms that can anonymise sensitive information automatically.
With RAG, businesses don’t need to share raw data with the model provider. Instead, they can process it internally first to ensure that only intended data is transmitted.
Avoiding hallucinations
As with the brain, whenever AI models are missing information, they tend to fill in the gaps to come up with an explanation – even if it’s not completely accurate.
Retrieval-augmented generation allows you to combine business documentation and data into the system with information from external sources. Having an extra source of knowledge retrieval is one of the best solutions we have against AI generating false information, known as a hallucination.
It is essential to ensure that outputs are grounded in real life, not guesses. With this fortification, teams have even more control of the data set the language model pulls from.
With less powerful language models, hallucinations are more common as the technology tries to make sense of unclear patterns and tries to reconcile any discrepancies.
Depending on the industry, businesses must know that hallucinated outputs could further exacerbate organisational security risks. Inaccurate data that is relied upon in society-critical areas could lead to poor decision-making, unauthorised access to systems, misallocation of resources, and inaccurate analyses.
Aspects like inaccurate analyses might also guide employees to act based on incorrect recommendations.
Optimising retrieval-augmented generation for trusted answers
Like any technique, refining RAG models is a continuous process. RAG tools are excellent at ingesting business documents and data that can give users relevant and specific responses.
However, it can be less effective if users don’t need a specific answer and instead look for all answers. For instance, if a user were to ask, ‘Tell me about our content on technical infrastructure’ versus ‘Show me a list of all of our design documents’.
To address this challenge, RAG models should be designed to recognise – and search by – a user’s intent.
With an understanding of intention, RAG tools can adapt responses based on the nature of each query and share the best-suited information.
Businesses can also introduce alternative ways to query data. At the moment, semantic search is the most common approach, but it can sometimes struggle with ambiguity or focus too much on matching each query precisely with its existing knowledge.
Teams can optimise semantic search by combining it with other methods, such as keyword-based search.
The generative AI sandbox
If businesses want to overcome their fear of AI in the future, they must take back control over the data being used to fuel it.
If leaders can implement ways to manage and own their data, they can better implement a sandbox system, like RAG, that allows teams to innovate safely – and in a way that fits existing business processes.