The DAEMON project, supported by the European Commission, lays the foundation to 6G systems that are AI-ready by design.
The vision for 6G mobile networks sets an extraordinarily high bar for mobile networks, which are expected to run entirely diverse classes of services, and do so with outstanding performance. Its near-zero latency, apparent infinite capacity, and 100% reliability and availability will make the communication infrastructure fully transparent to the applications. Meeting this ambitious goal requires mobile network architectures to become able to instantly orchestrate physical resources and Virtual Network Functions (VNF) across different network domains, in concertation with time-varying demands and multi-tenancy requirements.
Zero-touch technologies will be paramount in managing the substantially increased complexity of 6G networks, by fully automating control and orchestration operations and dramatically increasing the speed and effectiveness of decision-making. Therefore, the success of 6G will vastly depend on the quality of the Network Intelligence (NI) that will run at schedulers, controllers, and orchestrators across network domains.
What is the DAEMON project?
The DAEMON project1 aims to ensure that NI can operate optimally in future-generation mobile network infrastructures, by setting forth a considerate adoption of Artificial Intelligence (AI) models for NI, and a pragmatic and systematic integration of NI in 6G mobile networks. By doing so, DAEMON implicitly aims at ensuring that the NI drives zero-touch 6G systems towards meeting their very high expectations in terms of performance, sustainability, and reliability. Achieving this goal requires updating the network architecture so that it can best accommodate NI, as well as rethinking the design and deployment of Machine Learning (ML) solutions, tailoring them to increasingly complex and diversified mobile networking environments.
The overarching goal above can be deconstructed into three technical objectives.
Designing a ‘NI-native architecture’ for 6G systems
While present efforts for integrating NI in 6G mobile networks aim at tweaking Machine Learning solutions so that they fit networking environments, DAEMON upends the approach, and seeks to update the network architecture so that it natively supports NI operations. This objective stands on two pillars:
- Enhancing the current architectural vision of standardisation bodies so that it enables a
comprehensive co-ordination across the many NI instances that operate in the network infrastructure; and - Fostering a deeper and tighter incorporation of NI into the network infrastructure, by developing and demonstrating novel co-ordinated NI solutions that operate at the VNF level and are implemented in the user plane.
Establishing fundamental guidelines for a pragmatic design of NI
DAEMON challenges current practices in Machine Learning for network automation, which slightly adapt solutions developed in other computer science communities (e.g., in Machine Learning, or image processing) to the networking context. This conventional approach only yields a superficial integration of AI in the network architecture, and hence falls short of DAEMON’s vision of a NI-native 6G network. To ensure that NI can benefit to a maximum extent of recent advances in AI, DAEMON adopts a structured strategy for the definition of original AI models that are specifically tailored to NI tasks, by:
- Assessing when powerful but non-interpretable Deep Learning (DL) models are appropriate, and when statistical, analytical or hybrid models should be preferred;
- Devising custom and/or self-learned loss functions that overcome the largely unexplored ‘loss-metric mismatch’ problem in NI design; and
- Designing AI models that can adaptively trade off accuracy for network-critical metrics like computational complexity and execution times.
Developing specialised NI-assisted network functionalities for 6G mobile networks
DAEMON designates a concrete list of key network functionalities for which it will devise and implement NI algorithms capable of taking full advantage of the proposed NI-native architecture, and spanning varied planes, domains and operation timescales. Specifically, the project targets zero-touch operation of eight critical 6G network functionalities: (i) Reconfigurable Intelligent Surfaces (RIS) control, (ii) multi-timescale edge resource management, (iii) in-backhaul support for service intelligence, (iv) compute-aware radio scheduling, (v) energy-aware VNF placement, (vi) self-learning MANO, (vii) capacity forecasting, and (viii) automated anomaly response. These are critical functionalities that span control and user planes in diverse network domains, and run at timescales from milliseconds to hours. Tackling them jointly will allow for assessing the NI co-ordination capabilities of the DAEMON architecture, and demonstrating its advantages in terms of network performance, sustainability, and reliability.
Utilising Machine Learning to enhance the performance of 6G mobile networks
The joint achievement of the objectives above results in a re-thinking of the design and integration of Machine Learning solutions so that they are tailored to network environments, from multiple viewpoints.
First, it allows acknowledging and understanding the limits of AI for mobile networks: the activities of the project inherently carry out the first systematic, critical analysis of which NI problems can be appropriately solved with AI models rather than other techniques, contributing to the provisioning of a solid set of guidelines for the utilisation of Machine Learning in network management tasks.
Secondly, it paves the road to the design of practical NI algorithms empowered by highly customised AI techniques that finally respond to the specific needs of network management functionalities (e.g., latency guarantees, computationally constrained environments, limited training data availability, or unknown relationships between decision and performance, just to cite a few).
Thirdly, it goes beyond the current practice of designing NI solutions as if they had to operate in isolation. It recognises that different AI models need to co-exist in the same network environment, reciprocally affecting each other’s decisions. This leads to the design of a proper end-to-end NI-native architecture that, for the first time, enables the co-ordination of the many and varied NI instances deployed in the network.
Achievements of the DAEMON project
The DAEMON project is now in its third year of execution, and attained a number of major achievements, in line with the objectives listed above. We would like to bring attention to the following results in particular.
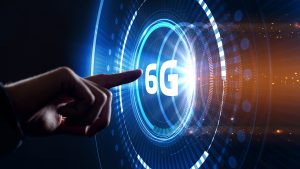
DAEMON developed a first design for an updated architectural model for 6G networks that are NI-native, i.e., support NI operations by design. The proposed model stems from an analysis of current standards and platforms proposed by leading organisations like 3GPP, ETSI or O-RAN, which showed how they have gaps in terms of: (i) mechanisms to co-ordinate intelligence across network micro-domains; (ii) solutions for decentralised and unified data management across NI instances; (iii) support for managing the NI lifecycle; and (iv) methodologies for the defining and representing of NI models. Our proposed model introduces a novel NI Plane (NIP) that complements those already existing in 5G architectures (i.e., user, control and management planes) and fills the gaps above. Details can be found in various notes and papers,2,3 and the DAEMON design has been adopted by the 5G Infrastructure Association in its 6G mobile networks white paper.4
DAEMON developed tens of NI algorithms that support the dependable and automated operation of a target set of eight key network functionalities, ranging from virtualised radio access resource scheduling5 to automated anomaly response,6 passing by, e.g., in-band inference7 and energy-aware VNF orchestration.8 These NI algorithms build upon and allow to validate guidelines for the design of network-specific Machine Learning models, and consists of tailored Deep Learning architectures as well as hybrid solutions combining the same with statistical modelling or optimisation tools.
DAEMON has produced outstanding scientific and industry impact during the first two years of execution. On the scientific side, the results produced by the project have been presented at most of the top-tier conferences in the networking domain, including ACM SIGCOMM, ACM MobiCom, IEEE INFOCOM (where the project contributed eight papers in three years), ACM CoNEXT, or ACM MobiSys. From the industry side, the project already generated five innovative patent applications, all of which have been selected for support by the Innovation Radar initiative of the European Commission. The project also had a significant impact on standardisation, with 39 contributions to SDOs that include O-RAN, ETSI and 3GPP.
The project has also developed, to date, four main demonstrators: (i) a new implementation of an O-DU that takes care of the encoding and decoding capabilities at 5G MAC and PHY levels, so as to integrate NI in vRANs beyond what is possible with the current O-RAN standard; (ii) a tool for anomaly detection based on federated learning in mobile edge environment; (iii) a solution to proactively trigger edge service relocation in order to maintain the level of service quality required by the end user; and, (iv) models for in-band inference at line rate in programmable user planes that have been showcased at Mobile World Congress 2023 in Barcelona and won a best demo award at the IEEE NetSoft 2023 conference.
Finally, it is worth mentioning that, while the project originally targeted low technology readiness levels (TRLs) for all the technical solutions to be developed, a few of the NI-assisted functionalities already achieved a TRL 5. Indeed, the DAEMON solutions for automated anomaly response for IoT devices and multi-timescale resource management are currently in the process of being validated via pilots in production environments, with the support of a global operator like Telefónica.
References
- DAEMON – Network intelligence for aDAptive and sElf-Learning MObile Networks, https://h2020daemon.eu/
- M. Camelo, M. Gramaglia, P. Soto, L. Fuentes, J. Ballesteros, A. Bazco-Nogueras, G. Garcia-Aviles, S. Latré, A. Garcia-Saavedra, M. Fiore, DAEMON: A Network Intelligence Plane for 6G Networks, 6GArch 2022, Rio de Janeiro, Brazil, Dec 2022
- L.E. Chatzieleftheriou, M. Gramaglia, M. Camelo. A. Garcia-Saavedra, E. Kosmatos, M. Gucciardo, P. Soto, G. Iosifidis, L. Fuentes, G. Garcia-Aviles, A. Lutu, G. Baldoni, M. Fiore, Orchestration Procedures for the Network Intelligence Stratum in 6G Networks, EuCNC & 6G summit 2022, Gothenburg, Sweden, Jun 2023
- M.K. Bahare, A. Gavras, M. Gramaglia, J. Cosmas, X. Li, O. Bulakci, A. Rahman, A. Kostopoulos, A. Mesodiakaki, D. Tsolkas, M. Ericson, M. Boldi, M. Uusitalo, M. Ghoraishi, P. Rugeland, The 6G Architecture Landscape – European perspective. Zenodo, Feb 2023
https://doi.org/10.5281/zenodo.7313232 - G. Garcia-Aviles, A. Garcia-Saavedra, M. Gramaglia, X. Costa-Perez, P. Serrano, A. Banchs, Nuberu: reliable RAN virtualization in shared platforms. ACM MobiCom 2021, New Orleans, USA, Oct 2021
- A. Lutu, D. Perino, M. Bagnulo, F.E. Bustamante. Insights from operating an IP exchange provider, ACM SIGCOMM 2021, Online, Aug 2021
- A.T.-J. Akem, M. Gucciardo, M. Fiore, Flowrest: Practical Flow-Level Inference in Programmable Switches with Random Forests, IEEE INFOCOM 2023, New York, USA, May 2023
- J.A. Ayala-Romero, A. Garcia-Saavedra, X. Costa-Perez, G. Iosifidis. EdgeBOL: Automating Energy-Savings for Mobile Edge AI, ACM CoNEXT 2021, Munich, Germany, Dec 2021
This document has been produced in the context of the DAEMON Project. The research leading to these results has received funding from the European Union Horizon 2020 research and innovation programme under grant agreement No.101017109.
Please note, this article will also appear in the fifteenth edition of our quarterly publication.