Professor Rizwan-uddin, Department of Nuclear, Plasma, and Radiological Engineering at the University of Illinois, reviews how new enabling digital technologies are aiding nuclear industry and nuclear engineering education.
Several enabling technologies have advanced very rapidly in recent years, allowing increasing levels of design, licensing, construction, and operational efficiencies in many fields. Educational institutions have played their role in the development of, and are now actively implementing modules, courses, and certificate and degree programmes to equip nuclear engineering graduates with these emerging tools. In some cases, education itself is also likely to benefit from them. In addition to those discussed in this article, there are also qualitative advances in ‘hardware-based’ technologies, such as 3D printing, robotics, and autonomous systems (hardware) that are changing the landscape of design, construction, and operations at a very rapid pace. However, these are not the subject of the current article. Our focus here is a broad overview of the recent advances taking place in the ‘digital’ world, revolutions being made possible by these advances, and the resulting challenges.
These enabling technologies are being developed and applied individually to address challenges facing the nuclear industry as well as collectively. Data analytics and Artificial Intelligence (AI)/ Machine Learning (ML) are being applied, for example, to fruitfully learn from historical data, and to help develop predictive maintenance models. Several universities and research laboratories have explored the use of Virtual Reality (VR)/Augmented Reality (AR) for the nuclear industry. It has been recognised that this technology is most useful in outreach, nuclear engineering education, and maintenance training – specifically for dose minimisation efforts. Technology advancement in VR is primarily driven by the video game industry. Final products for outreach or for training of NPP personnel look like a (training or educational) video game, which can be played on a laptop, tablet, smartphone, or in a 3D immersive and interactive environment (such as VR). If the recent introduction of VR for mass public consumption takes off, it stands to significantly advance the use of VR in the nuclear industry and in the field of nuclear engineering education.
Human-machine-interface
After the creation of the 3D model, development of specialised features relevant to the intended educational or training goals is the next major step towards the creation of a VR app. These features are usually the digital/virtual version of human-machine-interface (HMI) and corresponding actions. An action in the virtual space should result in the corresponding consequences of that action playing out in the virtual world. These consequences are determined or calculated using the corresponding physics models, and are displayed in various formats – including virtual LED displays, virtual computer monitors, and even virtual models of analogue metres. Scripts have been written, for example, to move a control rod in a reactor core and thus change the reactivity in the physics model as the virtual UP or DOWN button is clicked.
Interactivity (or the HMI) in these virtual models has been hardware-specific (mouse, keyboard, Xbox controller, and other controllers that are specific to particular VR devices). As yet, there is no standardisation among hardware developers to help ease the transition from one platform to another. Hence, as new hardware becomes available, old 3D models with interactive features need to be tweaked to map the interactive features on to the buttons, knobs, and motion- or sound-sensing capabilities of the new hardware.
How a radiation-shielding lab enhances nuclear engineering education
Among the numerous nuclear education VR models, one relevant to nuclear engineering is the radiation-shielding lab, in which students can conduct virtual experiments to determine the attenuation coefficient of different materials by measuring the transmitted radiation for different shielding block thicknesses. Fig. 1 shows a screenshot of the experiment on a computer screen. In another useful feature, a capability has been developed to display the given radiation level in 3D virtual models of a facility by displaying a (colour) radiation map on the floor. This capability has been coupled with the model of a virtual dosimeter feature in which – based on the radiation level, location of the player and time spent – a counter at the bottom of the screen shows the accumulated dose received. Combining some of the built-in features in most first-person games with the virtual dosimeter feature, it is a relatively easy extension to develop a dose-minimisation game in which a player is expected to complete a set of tasks in a radiative environment while minimising the dose received.
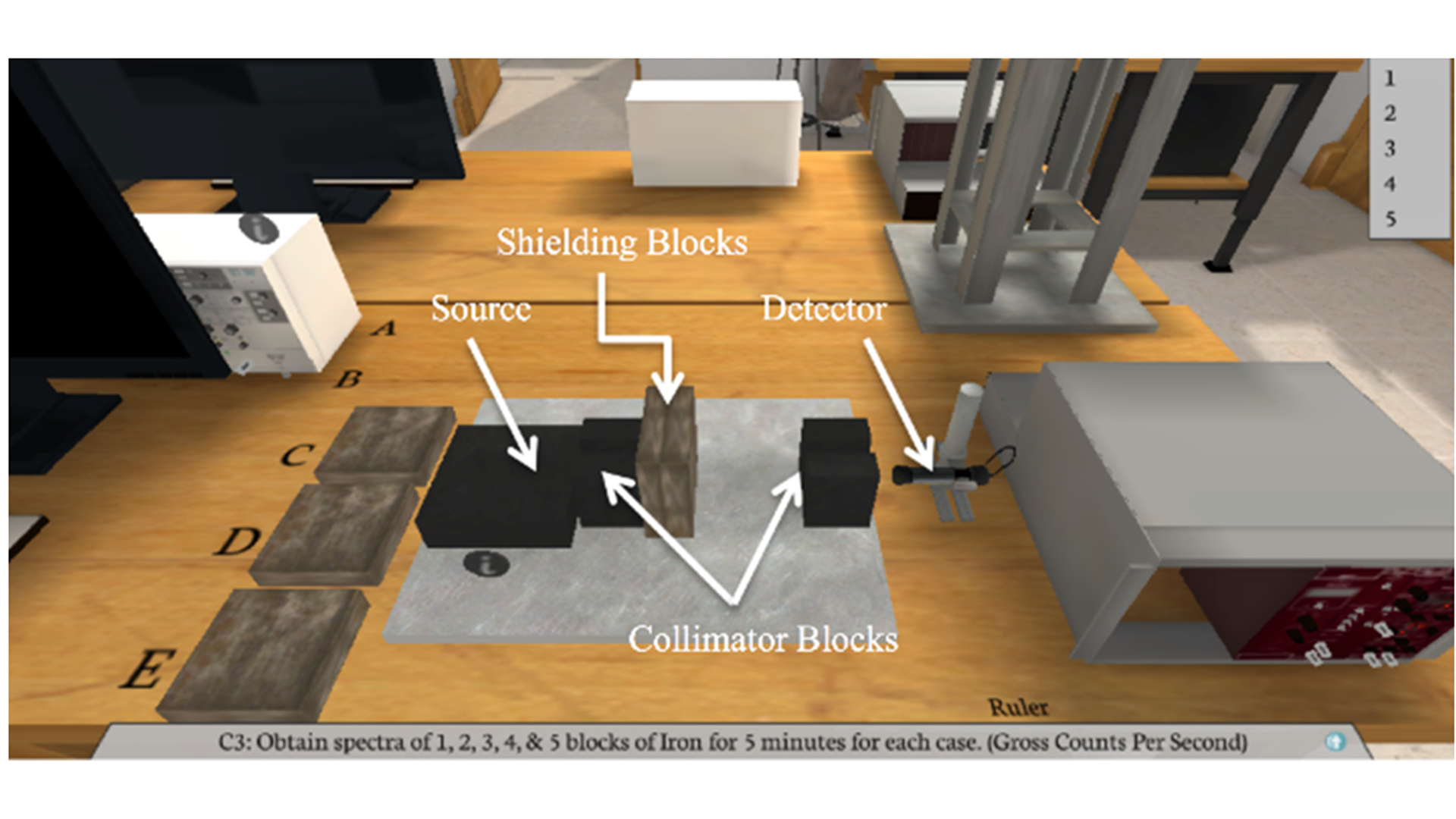
A detailed 3D model of a TRIGA research reactor facility, including its control room, is one of the many facilities developed. This model is a fairly accurate and to-scale representation of the TRIGA reactor facility that existed on the campus of UIUC until 2004, when it was decommissioned. This 3D model can be experienced on laptops, in 3D immersive VR devices, as well as on smartphones. A short-lived challenge with smartphone-based apps of these nuclear-specific applications was that they allowed very limited modes of interaction with the model. The challenge has been addressed by simply connecting the smartphone using Bluetooth with an Xbox controller, thus making available joysticks and several buttons to interact with the virtual model. Fig. 2 shows a picture of a user playing the dose minimisation game in the TRIGA facility on a smartphone using an Xbox controller. Extensions to AR and Mixed Reality (MR) applications are also being developed. These models and features are being implemented for nuclear engineering education and workplace training. Extensive implementation of VR models for education and training can then lead to large amounts of data that can subsequently be used to implement AI and ML models to, for example, operate robots autonomously.
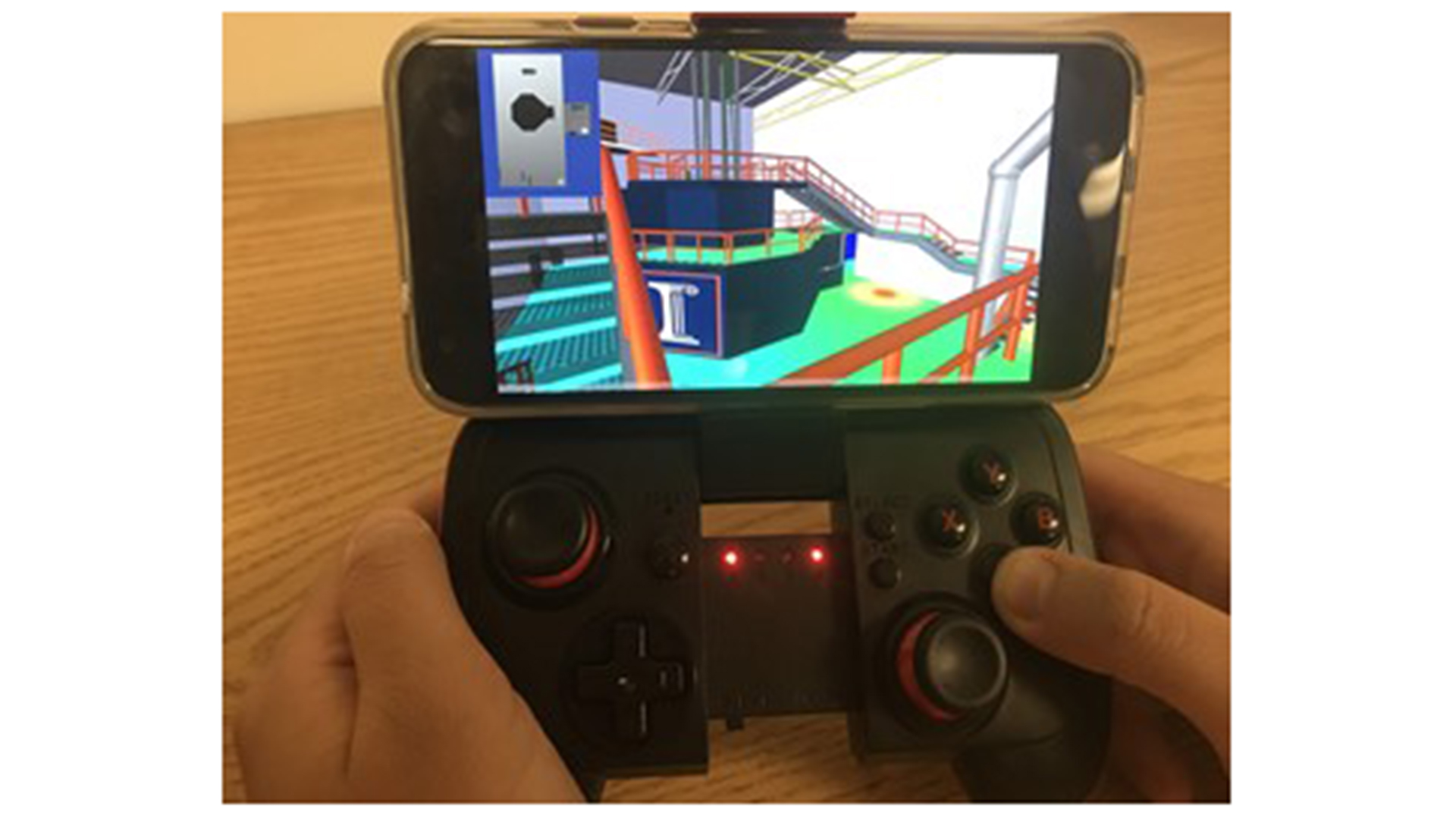
The role of digital twins in the nuclear industry
In many industries, collective application of these enabling technologies often are converging to what is being called a digital twin (DT). Articles with titles similar to ‘Rise of the digital twin’, or some slight variation of it, have recently appeared in the The Times, Forbes, and Wall Street Journal. Nuclear industry and researchers have also embraced DTs. Being relatively new, the exact definition of ‘digital twin’ is not agreed upon by all. Five panellists on a recent panel on digital twins, organised at a national meeting, felt not only the need to define what they meant by DT, but they also ended up defining DT in five different ways. The very term DT implies a digital system that mimics a physical asset, which is why the physical asset would not be considered to be a part of the DT. However, this often is not the case. DT is sometimes used to refer only to the digital equivalent of the physical asset, and sometimes DT refers to the collection of the physical asset and its digital equivalent. In this article, the two together will be referred to as the ‘DT System’. The former version of the DT, when there is as yet no physical asset, is generally used when DT is being developed for the purpose of improving the design of a future asset. In this case, there can be no sensory data from the physical asset feeding into the DT. This digital-only version of the DT is intended to be used to simplify and optimise the design before the asset is built.
To make ‘digital twin’ sufficiently distinct from ‘simulators’, the term DT in this article will be reserved for digital systems that mimic nuclear engineering systems, and also have data being measured in an associated hardware fed into the DT. DT is a digital asset that closely mimics the state of the physical asset. If, at some point in time, the state of the digital system does not match that of the physical system (possibly due to shortcomings in the simulation capabilities), the sensory data from the physical system is to be used to minimise the gap between the physical and digital asset’s states. To allow other uses of the term DT, ‘DT system’ will be used to refer to the collection of the DT and the corresponding physical asset, as well as any human machine interface. ‘DT for design’ will be used to refer to a DT developed during the design stage of a future physical asset.
Fig. 3 shows a schematic diagram of a Digital Twin System, consisting of a physical asset, its digital twin, and a third part that represents all-things-human. Even the humans who may physically be inside the physical asset are schematically inside the circle representing humans (Fig. 3). High fidelity simulations are an integral part and a necessity for DTs. High and low fidelity simulations are represented in the ‘simulator’ part of the digital twin. The flow of information, data, actions and recommendations, and sensory data are represented by arrows. The physical asset is expected to be highly instrumented. Operational history of the physical asset is also expected to be a part of the DT. Application of AI/ML are distributed over the DT as well as in the human part of the system.
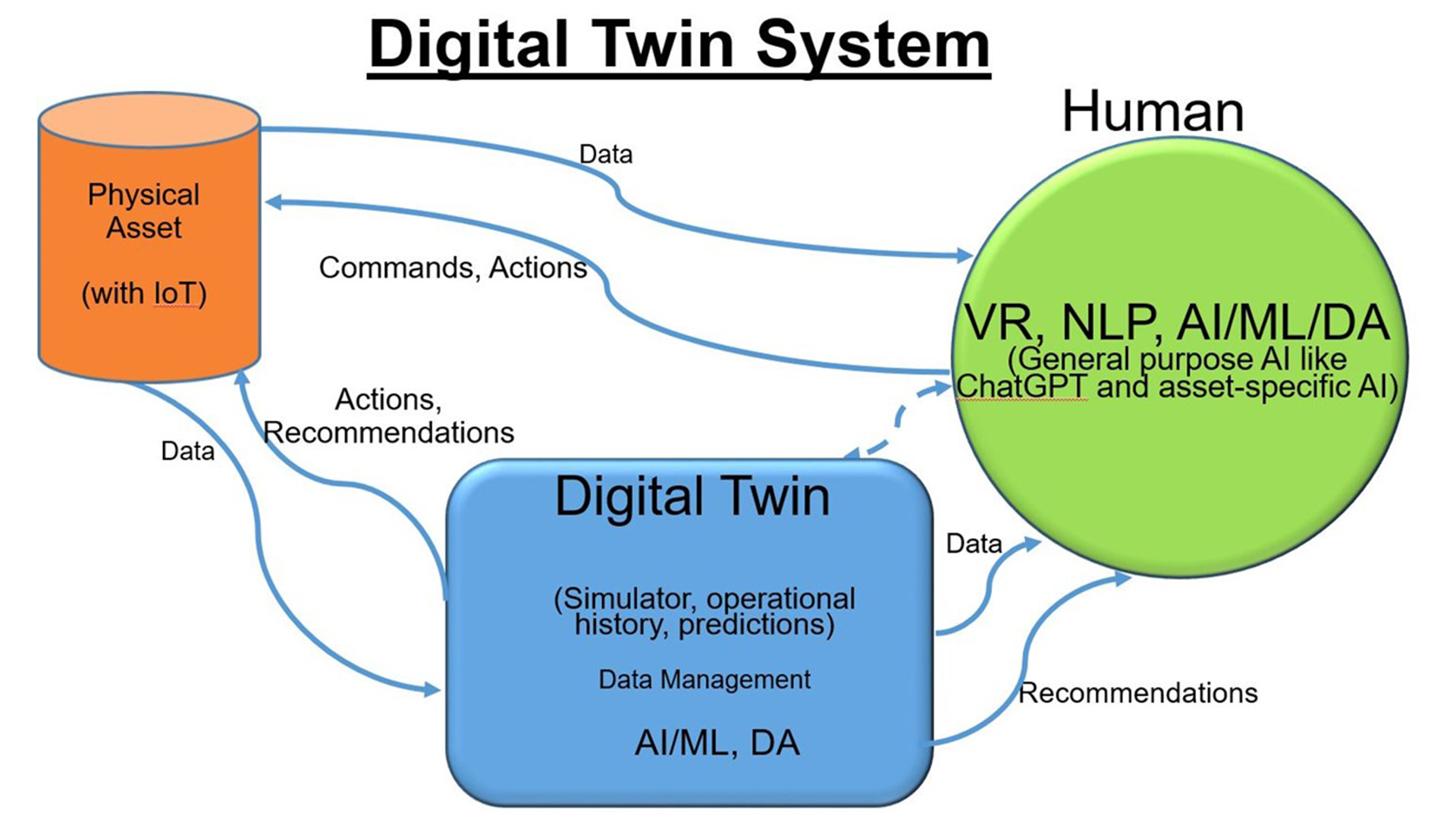
DTs seem to be the solution for many challenges facing the nuclear engineering industry today. These include: need for faster design iteration, faster licensing, autonomous operations and need for more efficient operation, leading to reduced O&M cost. Two of the most promising and near-term applications of the digital twins in the nuclear industry are to help the transition, 1) from hands-on to autonomous control, and 2) from planned to predictive and risk-informed maintenance. Both of these are aimed at reducing the operations and maintenance cost. A ten-fold reduction in O&M cost is envisioned in a U.S. Department of Energy (DOE)-sponsored research initiative under the Advanced Research Projects Agency-Energy’s (ARPA-E’s) Generating Electricity Managed by Intelligent Nuclear Assets (GEMINA) programme. Much of this programme is aimed at new reactor development, and hence a finished physical asset does not yet exist. Therefore, many of the digital twins being developed under this programme are ‘digital twins for design’. These DTs for design will eventually expand to ‘DT systems’ once the physical asset has been built, and sensory data starts getting fed into the DT. Taking advantage of system performance and data from excessively monitored physical assets fed into data analytics, AI and ML systems will lead to early warning, and improved diagnostics, and prognostics.
Early case studies of DTs in the nuclear industry include Idaho National Lab’s prototype digital twin of two physical assets: Single Primary Heat Pipe Extraction and Removal Emulator (SPHERE) and Microreactor AGile Non-nuclear Experimental Testbed (MAGNET). This testbed is intended to demonstrate remote and autonomous operations. Heat in MAGNET is generated using electricity. The DT of MAGNET consists of a model of the physical system, and it is fed sensory data from the physical asset. ML and AI techniques are being used to predict performance and to demonstrate autonomous operations.
ChatGPT
The next frontier in this space is expected to be a marriage between DT (encompassing traditional simulations with AI/ML/DA/VR and sensory data) with intelligent natural language processing (NLP) systems, such as ChatGPT. Given the storm ChatGPT has caused in the first three months after its release, and ways in which it is already being used, any attempt to predict its long-term impact is likely to fall short. In the context of topics of this article, it is, however, easy to visualise DTs being available to human operators (possibly at locations far from the physical assets) in the VR environment, linked with knowledge management systems comprising of general-purpose systems such as ChatGPT, as well as specialised (maybe even proprietary) knowledge bases specific to the physical asset. Information extraction would happen via spoken commands. The general-purpose and system-specific knowledge bases would intelligently integrate responses to specific commands before passing it to the humans. Responses could be in voice or text form. When appropriate, this hybrid, intelligent system can also make ranked recommendations for actions along with associated costs, benefits, and risks. This information can be made available through VR or in AR HMIs. Voice command and voice responses are likely to become much better integrated with query-and-responses than they currently are. In addition to responses to specific queries, smart AI-based NLP systems can also be used to make recommendations on their own (without a query), in text or voice form, based on continuous analysis of sensory data processed through the AI and data analytics parts of the system.
Cybersecurity issues posed by digital technologies
Arguably, the biggest challenge in these digital technologies is cybersecurity. As increasingly more decisions are made by AI systems, it is easy to visualise situations in which the decision-making process is taken over by a malicious player, or the AI-based decision-making process is fooled by taking over the digital sensory input process and feeding malicious sensory data, thus making the system itself take actions that are not compatible with the actual state of the physical asset. A simple example is that of a self-driven car whose environment-detecting sensors (LiDAR) are compromised. In the case of a nuclear reactor, data from the temperature sensors, position of the control rods, and coolant flowrate metre, if compromised and altered, can potentially fool the automatic decision-making system. While new digital assets make available additional cyber pathways for attacks, advances are continuously being made in cybersecurity to protect these digital and physical assets. One of the goals of the ongoing research at Idaho National Lab on DTs is to use digital systems to help create a reliable and secure multi-layered (cyber) security system – the very source of vulnerability is also the tool that is likely to plug-in that vulnerability hole.
AI/ML, VR/AR, NLP and digital twins are changing the landscape of technology, industry, and business. Their full potential is yet to be realised.
Please note, this article will also appear in the thirteenth edition of our quarterly publication.