Researchers have used machine learning techniques to massively accelerate the search for Parkinson’s treatment.
Researchers from the University of Cambridge designed and used a machine learning-based strategy to identify compounds that block the clumping, or aggregation, of alpha-synuclein, the protein that characterises Parkinson’s.
The technique was used to quickly screen a chemical library containing millions of entries and identify five highly potent compounds for further investigation.
The research, ‘Discovery of potent inhibitors of α-synuclein aggregation using structure-based iterative learning,’ was published in Nature Chemical Biology.
Speeding up screening processes for developing Parkinson’s
Parkinson’s affects more than six million people worldwide, with that number projected to triple by 2040. However, no disease-modifying Parkinson’s treatment is currently available.
This has been a major obstacle in Parkinson’s research because of the lack of methods to identify the correct molecular targets and engage with them.
This technological gap has severely hampered the development of effective treatments.
Screening large chemical libraries for drug candidates—which needs to happen well before potential treatments can be tested on patients—is enormously time-consuming, expensive, and often unsuccessful.
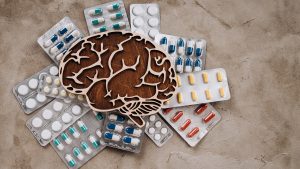
Using machine learning, the researchers were able to speed up the initial screening process tenfold and reduce the cost by a thousand-fold, which could mean that potential Parkinson’s treatments could reach patients much faster.
“One route to searching for potential treatments Parkinson’s treatment requires the identification of small molecules that can inhibit the aggregation of alpha-synuclein, a protein closely associated with the disease,” said Professor Michele Vendruscolo from the Yusuf Hamied Department of Chemistry, who led the research.
“But this is an extremely time-consuming process – just identifying a lead candidate for further testing can take months or even years.”
How the machine learning method was tested
The Cambridge team developed a machine learning method in which chemical libraries containing millions of compounds are screened to identify small molecules that bind to the amyloid aggregates and block their proliferation.
A small number of top-ranking compounds were then tested experimentally to select the most potent inhibitors of aggregation.
The information gained from these experimental assays was fed back into the machine learning model iteratively, and after a few iterations, highly potent compounds were identified.
“Instead of screening experimentally, we screen computationally,” said Vendruscolo.
“Using the knowledge we gained from the initial screening with our machine learning model, we were able to train the model to identify the specific regions on these small molecules responsible for binding. Then, we can re-screen and find more potent molecules for Parkinson’s treatment.”
She added: “Machine learning is having a real impact on the drug discovery process – it’s speeding up the whole process of identifying the most promising candidates.
“For us, this means we can start work on multiple drug discovery programmes instead of just one.”