Using Machine Learning, federated health data, and a unique approach to shared value, the IMI SOPHIA project is changing the narrative and inspiring a new future for obesity care.
What do we know about obesity? Obesity currently affects 150 million people in Europe and 650 million people worldwide, according to the World Health Organization (WHO).1 This number has been steadily rising, surpassing the WHO’s global targets and increasing the risk of associated health concerns such as type 2 diabetes, gallbladder disease, liver disease and increased cardiometabolic and cancer risk.2–4
Achieving better health outcomes requires new knowledge and understanding of the biological underpinnings of obesity. By analysing data from large-scale cohorts and conducting in-depth qualitative studies of those living with obesity, the SOPHIA project aims to predict complications, target treatments better, and change the narrative around obesity to make it more equitable and patient centric.
Professor Carel le Roux, co-ordinator of SOPHIA and obesity physician at the Diabetes Complications Research Centre at University College Dublin, said: “Our mission within SOPHIA is to enable healthcare professionals to reliably predict the complications of obesity and who will respond to treatment.”
The SOPHIA project (Stratification of Obesity Phenotypes to Optimise Future Obesity Therapy) is a public-private partnership funded by the Innovative Health Initiative (IMI) in collaboration with the European Commission. The SOPHIA project is part of a momentum shift in the study of obesity.
It brings together partners from industry, academia, and patient and public organisations to provide better tools for the stratification of people with obesity according to disease risk and treatment response and to meet the needs of future healthcare systems.
Not everybody has the same risk for a given BMI
When examining two patients with the same body mass index (BMI) and adiposity distribution, one may have type 2 diabetes while the other may have no metabolic complications but significant functional impairment. Moreover, one may respond extremely well to bariatric surgery, while the second may not.
Daniel Coral of the Lund University Diabetes Centre in Sweden, said: “We (used to) think of the relationship between obesity and type two diabetes as always increasing simultaneously. What has been shown is that a lot of individuals don’t have the metabolic risk for their given BMI, including the risk of type 2 diabetes.”
By diagnosing and treating well-defined subpopulations of obesity, we can achieve a greater benefit with fewer side effects and lower costs to healthcare systems.5 The work within the SOPHIA project is taking us closer to defining who will experience adverse complications – and who might respond best to specific interventions.
Changing the narrative – beyond BMI
Patients are currently defined by BMI or waist measurement – based on current clinical and regulatory guidelines – which leads to obesity subtypes being treated as if they were identical. Using better-defined subpopulations, we will be able to select participants who are likely to progress most rapidly through the disease process or who are likely to respond well to the targeted intervention.
We must move past the ‘one size fits all’ approach, as this has not yielded the desired results. Throughout our work, it has become clear that stakeholders share many of the same concerns and barriers to adopting innovative solutions to obesity management across healthcare systems. It is also clear that there is a lack of clarity for specific terminology in the language of obesity as a chronic disease.
Our work with patients, healthcare professionals, policymakers, researchers, industry and payers (social insurers) across 18 nationalities has found consensus for statements that refer to obesity as a chronic, relapsing disease with many root causes as well as factors that affect its progression, including genetic, environmental, and (epi)genetic factors.6
In March 2021, the European Commission issued a brief defining obesity as a ‘chronic relapsing disease, which in turn acts as a gateway to a range of other non-communicable diseases.’ This was largely thanks to the advocacy work in SOPHIA and our work with the European Association for the Study of Obesity (EASO).
“A number of gaps to implementation are now very clear,” said Jacqueline Bowman-Busato, SOPHIA partner and EU Policy Lead at EASO, “much remains to be done to design and deliver meaningful national plans for the prevention, management and long-term treatment of obesity.”
Obesity as a set of chronic, complex diseases
There are many discrete subpopulations of people with different types of obesity.8 Defining these subtypes through phenotyping is a major step forward in understanding obesity. It is, however, possible that there are subclusters within each cluster. In short, the work is just beginning.
In contrast to being considered a risk factor for other diseases or a failure of self-control, obesity is more likely itself a set of chronic, complex diseases.7 The work of the SOPHIA project within this field is to remove the stigmatising declaration to ‘eat less and move more’ and advance the growing body of evidence finding that obesity is the result of a complex pathogenesis with a biological basis.
Although the health risks associated with obesity have been well documented, it is equally important to include perspectives from those who live with this complex and chronic disease. The association between excess adiposity and increased impairment in activities of daily living shows the need for research that includes the patient experience.8
Including the patient voice
Historically, patients were positioned passively on the receiving end of healthcare and policy.9 A systematic review and synthesis by SOPHIA researchers highlighted several studies where stigma and negative treatment were perpetuated by healthcare providers, particularly regarding the stigma around weight loss surgery, which was seen by some as ‘taking the easy way out.’ Unsurprisingly, we also see a lack of understanding regarding obesity as a disease, available treatment pathways and a strong sense of personal responsibility.10
Hilary Craig of the University College Dublin in Ireland, said: “Understanding patient preferences for patients with obesity complications may impact treatment planning to make interventions more effective. This may improve patient engagement in treatment adherences and can reduce costs as patients become more compliant with treatment.”
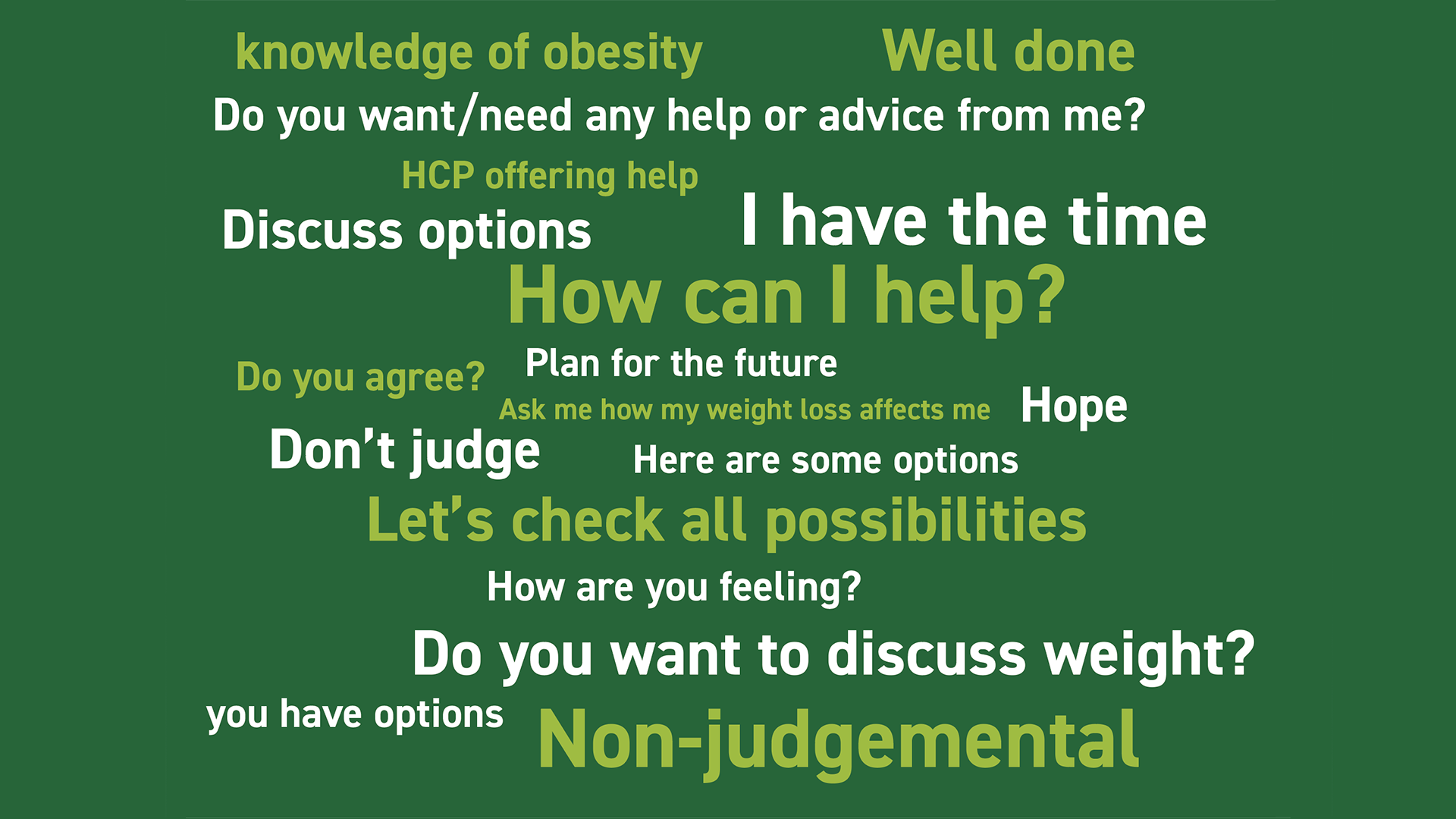
Bridging data silos and GDPR requirements
For legal and ethical reasons, performing analysis by pooling individual-level data from different clinical studies in a central repository is not feasible. This challenge could limit the potential of combined data for biomarker discovery and disease phenotyping.
In contrast to traditional analysis, which is often performed on a single server, the SOPHIA project put in place a federated analysis system that performs calculations on multiple servers in parallel. The SOPHIA federated database is built on open-source software and a privacy-preserving data framework that allows researchers to analyse sensitive data without physically moving the data to a central repository.11
Carl Delfin, Postdoctoral researcher at Novo Nordisk in Denmark, said: “It is really astounding that you can analyse datasets across Europe without a single datapoint ever leaving their respective node. “Being able to access, query, and analyse different types of data without leaving our own computers – and without compromising privacy or GDRP compliance – has been immensely beneficial for SOPHIA.”
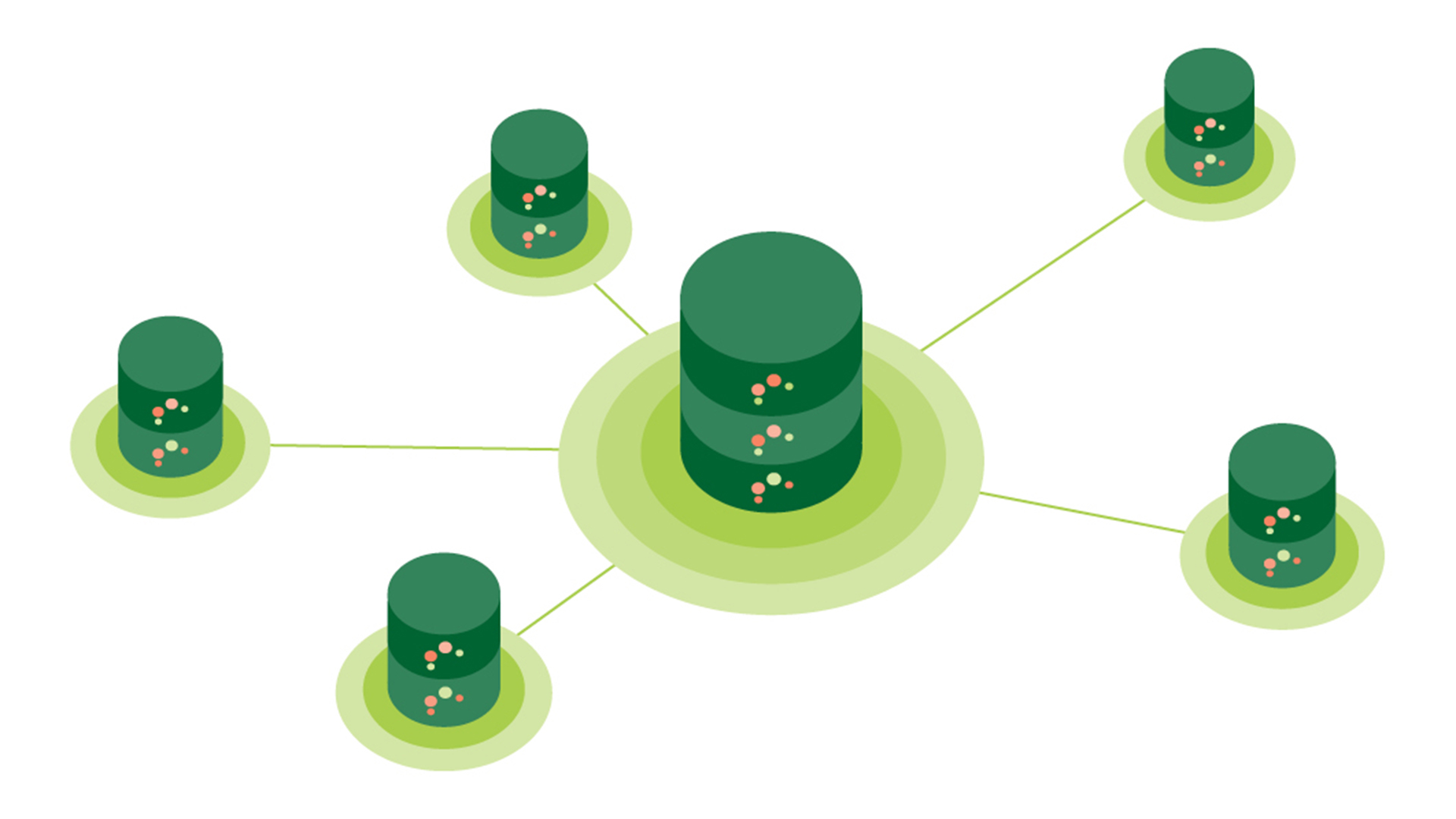
The result is a federated database with ten nodes and 18 clinical cohorts accessible via a central gateway, where the original cohort data never leaves the collaborating node. As any new data can be harmonised and connected to the federated database, this solution is highly scalable, and as more datasets are included in the federated database, the more powerful it can become for analysis and prediction.
Mark Ibberson at the Swiss Institute of Bioinformatics in Switzerland said: “In the future, I believe that most data analysis will be performed on federated system. This is a fast-moving field where technologies are constantly evolving with new possibilities for performing advanced AI on remote data.”
Can we cluster people into different obesity subgroups to better predict future risk?
Even when BMI is comparable, lean and fat mass distributions often vary from one person to the next.12 Using UMAP, an algorithm for dimension reduction based on manifold learning techniques and topological data analysis, we are trying to understand what percentage of the population of people with the disease of obesity have discordant biomarkers, where at least one biomarker measurement deviated considerably from the expected level, given their BMI.
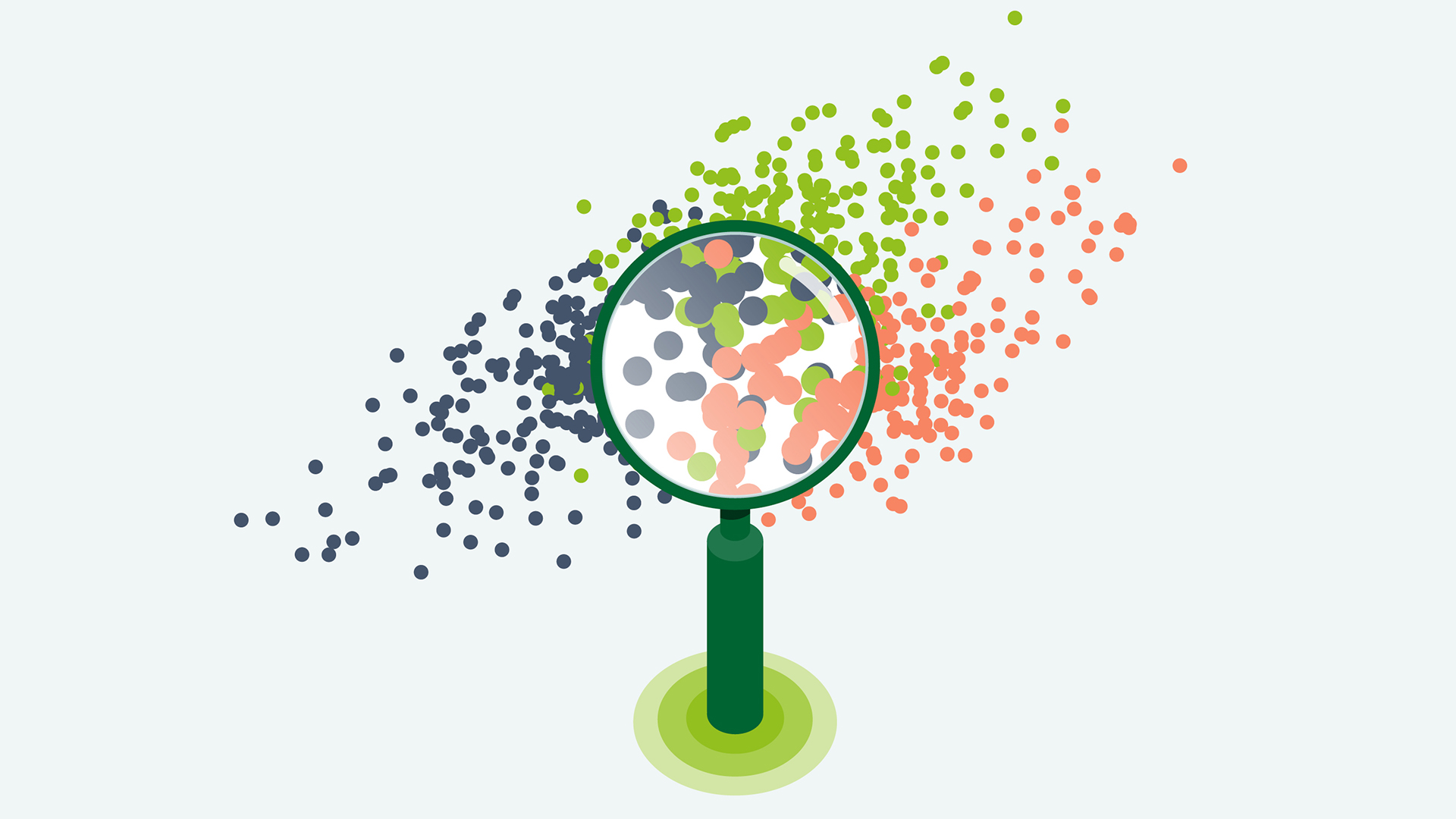
The discordant clusters may have other important differences in disease progression compared to the concordant cluster. For example, a cluster characterised mainly by glucose levels had more type 2 diabetes cases than the concordant cluster, even after adjusting for glucose levels.
Daniel Coral, Doctoral student at Genetic and Molecular Epidemiology at Lund University in Sweden, said: “Subclassifying diseases helps us understand better the diverse mechanisms through which these diseases arise and why some individuals display unexpected phenotypic patterns of enhanced susceptibility or resilience. This can translate into better diagnosis and treatments than we currently have for many diseases.”
What could these clusters tell us?
For example, the discordant hyperglycaemic cluster (DHG) may be linked to higher cardiometabolic risk. There may be a higher prevalence of self-reported type 2 diabetes and a higher risk of major adverse cardiovascular events (MACE). This risk may not be captured by traditional models that do not consider cluster membership.
Future research in the project will continue to consider the UMAP location of the individuals entering a clinical trials and locations with higher risk of adverse outcomes, such as major adverse cardiovascular events (MACE) and cardiovascular disease (CVD). When interventions are given, we may be able to determine where individuals shift on the UMAP projection and whether they move out of high-risk areas.
Using AI to predict weight loss trajectories
The SOPHIA project used data from patients, a Machine Learning model was developed that provides accurate individual weight trajectories expected during five years after bariatric surgery based on seven simple preoperative variables: age, weight, height, smoking history, type 2 diabetes status and duration, and the type of intervention.13
The model is incorporated into an easy-to-use and interpretable web-based tool. The project also showed the effect of diabetes duration and smoking, which were not previously included in weight loss surgery prediction models.
“The tool will substantially reduce uncertainty for patients as they can now make a much more informed decision about which surgical option to select. This is why we developed and validated this easy-to-use tool to predict an individual’s five-year weight loss after the most common bariatric operations,” said lead author Professor Francois Pattou, Lille University, France. “Patients and clinicians can now make more informed decisions.”
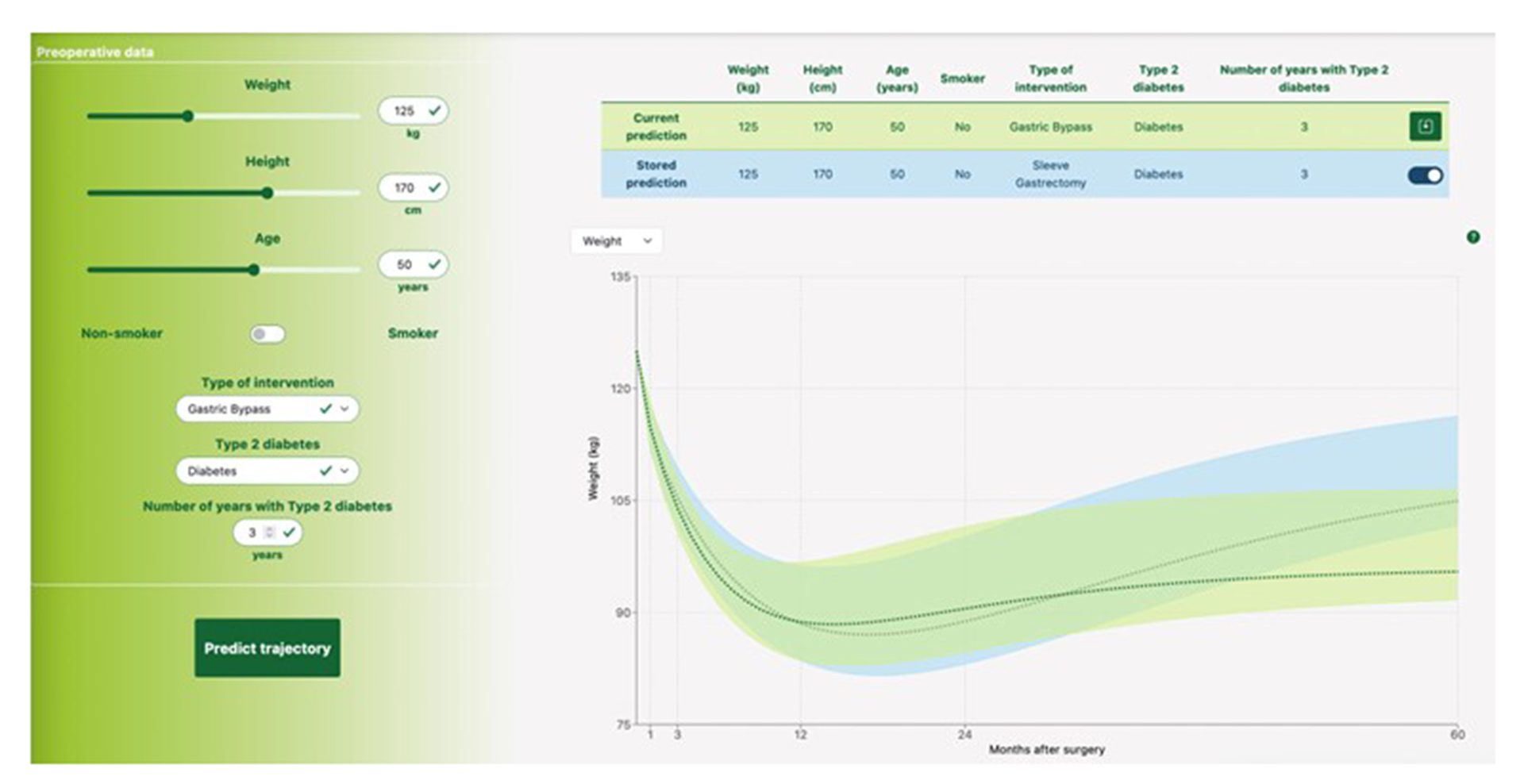
Our vision for the future of obesity care
The SOPHIA project is committed to adopting a new narrative around obesity and championing this approach in the broader community. We need to enable patients to understand that obesity is not their fault, but rather a subset of diseases with a biological basis.
The field of obesity has never evolved this quickly, and our approach to obesity will change fundamentally soon. The challenge of SOPHIA is not only the subsets of obesity phenotypes but also the needs and challenges of stakeholders and how we chart potential pathways at the intersection of improved patient care, more effective interventions, and reduced societal burden.
Our future vision is that individuals can be prioritised based on specific obesity and the associated potential for risk and health impact. Subsequently, they can be given targeted interventions to prevent or reverse their disease burden. This will take us one step closer to identifying the right obesity treatment for the right patient at the right time – and transform the approach to obesity care and management worldwide.
About SOPHIA
This project has received funding from the Innovative Medicines Initiative 2 Joint Undertaking (JU) under grant agreement No 875534. The JU receives support from the European Union’s Horizon 2020 research and innovation programme and EFPIA and T1D Exchange, JDRF, and the Obesity Action Coalition.
About Innovative Health Initiative (IHI)
IHI, formerly the Innovative Medicines Initiative (IMI), is the world’s largest Public-Private Partnership framework within health technology and life sciences. IHI is funded through the European Commission and aims to translate scientific knowledge into people-centred, cost-effective interventions responding to unmet public health needs. Visit www.ihi.europa.eu
References
- World Health Organization Key Facts about Obesity and Overweight. 2023 [cited 2023 Oct 18].
- Longo M, Zatterale F, Naderi J, Parrillo L, Formisano P, Raciti GA, et al. Adipose Tissue Dysfunction as Determinant of Obesity-Associated Metabolic Complications. Int J Mol Sci. 2019 May 1 [cited 2023 Oct 22];20(9).
- Aune D, Norat T, Vatten LJ. Body mass index, abdominal fatness and the risk of gallbladder disease. Eur J Epidemiol. 2015 Sep 30 [cited 2023 Oct 22];30(9):1009–19.
- Abdullah A, Peeters A, de Courten M, Stoelwinder J. The magnitude of association between overweight and obesity and the risk of diabetes: a meta-analysis of prospective cohort studies. Diabetes Res Clin Pract. 2010 Sep 1 [cited 2023 Oct 22];89(3):309–19.
- Tahrani AA, Panova-Noeva M, Schloot NC, Hennige AM, Soderberg J, Nadglowski J, et al. Stratification of obesity phenotypes to optimize future therapy (SOPHIA). Expert Rev Gastroenterol Hepatol. 2023 Oct 6 [cited 2023 Oct 22].
- Jepsen CH, Bowman-Busato J, Allvin T, Arthurs N, Goossens GH, Govers E, et al. Achieving consensus on the language of obesity: a modified Delphi study. EClinicalMedicine. 2023 Aug 1 [cited 2023 Oct 26];62:102061.
- Schwartz MW, Seeley RJ, Zeltser LM, Drewnowski A, Ravussin E, Redman LM, et al. Obesity Pathogenesis: An Endocrine Society Scientific Statement. Endocr Rev. 2017 Aug 1 [cited 2023 Oct 22];38(4):267–96.
- Blundell JE, Dulloo AG, Salvador J, Frühbeck G. Beyond BMI–phenotyping the obesities. Obes Facts. 2014 Nov 13 [cited 2023 Oct 22];7(5):322–8.
- Craig HC, Alsaeed D, Norris S, Holian J, Kennedy | Cormac, Feldman A, et al. Patient perspectives about treatment preferences for obesity with complications. Obes Sci Pract. 2023 Oct 29 [cited 2023 Oct 30].
- Farrell E, Hollmann E, le Roux CW, Bustillo M, Nadglowski J, McGillicuddy D. The lived experience of patients with obesity: A systematic review and qualitative synthesis. Obesity Reviews. 2021 Dec 1 [cited 2023 Oct 23];22(12):e13334.
- OMOP Common Data Model. [cited 2023 Oct 23].
- Goossens GH. The Metabolic Phenotype in Obesity: Fat Mass, Body Fat Distribution, and Adipose Tissue Function. Obes Facts. 2017 Jul 1 [cited 2023 Oct 23];10(3):207–15.
- Saux P, Bauvin P, Raverdy V, Teigny J, Verkindt H, Soumphonphakdy T, et al. Development and validation of an interpretable machine learning-based calculator for predicting 5-year weight trajectories after bariatric surgery: a multinational retrospective cohort SOPHIA study. Lancet Digit Health. 2023 Oct 1 [cited 2023 Oct 26];5(10):e692–702.
Please note, this article will also appear in the sixteenth edition of our quarterly publication.